|
| A perspective on the next challenges for TTS research | |
| |
Автор | Сообщение | A perspective on the next challenges for TTS research |
---|
mia V.I.P.
Сообщений : 184
Репутация : 7
 | mia | :: Чт Мар 05 2009, 20:55 |
Чт Мар 05 2009, 20:55 | |
| A perspective on the next challenges for TTS research J. Schroeter, A. Conkie, A. Syrdal, M. Beutnagel, M. Jilka, V. Strom, Yon - Jun Kim, Hong-Goo Kang, D. Kapilow AT & T Labs - Research
Abstract The quality of speech synthesis has come a long way since Homer Dudley's "Voder" in 1939. In fact, with the wide - spread use of unit-selection synthesizers, the naturalness of the synthesized speech is now high enough to pass the Turing test for short utterances, such as voice prompts. Therefore, it seems valid to ask the question "what are the next challenges for TTS Research? "This paper tries to identify unsolved issues, the solution of which would greatly enhance the state of the art in TTS. 1. Introduction Text-to-Speech (TTS) has come a long way from being an essential tool for a small group of important users, mainly for the handicapped, to delivering high quality synthetic speech for many other applications, such as in voice - enabled telecom services and on the desktop. Today's key TTS applications in communications include: voice rendering of text-based messages such as email or fax as part of a unified messaging solution, as well as voice rendering of visual / textual information (eg, web pages). In the more general case, TTS systems provide voice output for all kinds of information stored in databases (eg, phone numbers, addresses, car navigation information) and information services (eg, restaurant locations and menus, movie guides, etc.). Ultimately, given an acceptable level of speech quality, TTS could also be used for reading books (ie, Talking Books) and for voice access to large information stores such as encyclopedias, reference books, law volumes, etc., plus many more. Today's much larger set of viable applications for TTS technology is mainly due to the significant improvements in naturalness of the synthetic speech that unit-selection synthesis has made possible. A cursory analysis of the circumstances why TTS has gone "main-stream" might lead to the false conclusion that TTS is now "good enough" and that TTS Research has done its job. This paper is slated to dispel this notion by showing where current TTS technology falls short of its promises and where more research is needed. In the following, we will summarize the use of TTS in voice enabled telecom services as one important application, before outlining challenges in all important modules (from "text" to "speech") in unit-selection TTS. TTS for voice-enabled telecom servicesTTS is becoming an important part of voice-enabled telecom services. Such an application is depicted in Fig. 1. 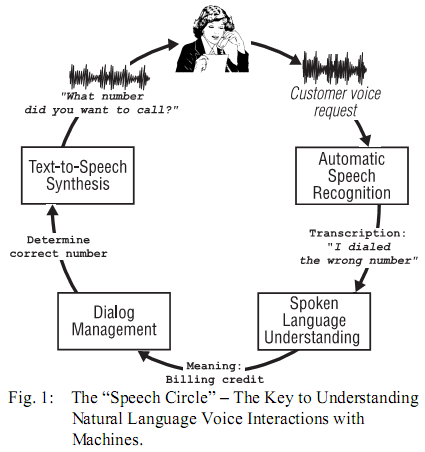 The speech signal related to a customer's voice request is analyzed by the subsystem shown on the top right. The decoded words are input into the Spoken Language Understanding (SLU) component. The task of the SLU component is to extract the meaning of the words. Here, the words "I dialed a wrong number" imply that the customer wants a billing credit. Next, a Dialog Manager determines the next action the customer-care system should take ( "determine the correct number") and instructs the TTS component to synthesize the question "What number did you want to call? " What about concatenative prompt generation? Such systems use recorded carrier phrases and fill in open slots such as names, times, dates, from other recordings. An example would be the prompt "Your flight from <Newark> To <Paris> leaves at <12:45 pm>, "where the <> bracket the slots to be filled from an inventory of allowed "Fillers" that also have been pre-recorded. Such concatenative systems clearly play an important role in high-quality (as perceived by the user), yet "simple" Natural Language Dialogue systems (mainly in system - initiative, form-filling, applications). Even for these simple applications, concatenative systems are not easy to design. The most difficult problem is to create fillers that match the required prosodic context. Note that a high - quality TTS system would do this "automatically" while - At the same time - being much more versatile and lower cost than a traditional concatenative system. For example, creating "static" prompts using a top-of-the-line TTS system in lieu of recording a voice talent can significantly shorten development time for a new voice - enabled service. Furthermore, TTS will be absolutely crucial for rendering highly customized voice prompts that will be created using dynamic information. With the accelerating developments in Natural Language technologies, TTS has moved from a "necessary evil "to the position of a" must-have ". The reason for this development is a movement away from rigid "system initiative "systems to" mixed initiative ", (ie, open) dialogue technologies that no longer allow one to record all possible prompts the system will ever play to its users. Today's systems are delivering highly tailored, up-to-the - minute, messages that have to be rendered by TTS for voice access (eg, via telephone, web, PC, PDA, etc.). Voice customization options for speech output via TTS The best-in-class TTS vendors today can now create a new, high-quality, TTS voice in about a month. This capability allows for three distinct ways of customization: • Voice fonts, a library of voices to choose from when adding TTS capabilities to any application, • Voice icons, exclusive, custom-developed TTS voices to extend corporate image, • Special domain voices, exclusive or non-exclusive, domain-specific TTS voices that result in extra-high quality synthetic speech for targeted applications domains (such as "travel"), and personalization (such as "soothing" to calm an unhappy customer). In the following, we will examine some of the challenges that still are largely unmet even in best-of-breed TTS systems and, consequently, require further research. Front-end challenges The task of a TTS "front-end" is to perform text normalization, word pronunciation, prosody prediction, and grammatical and semantic analysis. The purpose of such analyses is to predict what should be said and how it should be said in order to match human rendering. The output of this process is the input text tagged with symbolic information such as a list of phonemes, each with a set of features such as duration and pitch. Text normalization is the expansion of text into literal word tokens for items like measurements ( "... is 56 in. long "), currencies (" $ 4.5 billion "), and times and dates ( "9:20 pm on 9/15/2002"). Text normalization encom - passes abbreviation expansion that employs either a finite set of known mappings ( "Dr. F. Smith lives on Miller Dr. "), Or a totally open class of highly ambiguous map - pings "invented" by the creator of the text or part of a domain-specific jargon ( "The VOT is 3 ms." VOT = "Voice onset time"). In general, the challenge in text normalization lies in the fact that it is highly context - sensitive, language-specific, and application-specific [1]. Consequently, for extra-high quality, text normalization needs to be customized much like the voice recordings. Word pronunciation maps from orthography to phonemes. Again, the mapping is context-sensitive [2]. Consider the following examples: "lives" "it lives" vs. "nine lives" "bass" "bass boat" vs. "bass fiddle" "bow" "bow down" vs. "bow and arrow" "record" "world record" vs. "play and record" TTS front-ends usually employ a mix of pronunciation dictionaries and morphological analyses to avoid having to store all variants of a word in the dictionary, plus letter-to - sound rules as a fallback. Word pronunciation is difficult for names (people whose names are spelled the same disagree on the pronunciation; rules for name pronunciation might not match the rules for the rest of the language), and names are difficult to detect in running text. ( "Begin the work now!" Vs. "Begin met the US President. ") Again, in order to perform well, word pronunciation has to be customized for a given domain. Note also that there are also pronunciation-related challenges with the automatic labeling (section 5) done for creating TTS voices, the most obvious one being speaker - specific pronunciations and pronunciation inconsistency for words across different recording sessions. Prosody prediction is the assignment of speech melody, rhythm, and pauses to a given input text. Prosody conveys both syntactic and semantic information. Human speakers tend to break word streams at major syntactic boundaries in order to group words to meaningful chunks, but also because they have to breathe. The challenge is to approximate a syntactic parse well enough for this task. Furthermore, human speakers tend to make words sound also more prominent the more important information they carry. Here the challenge is that flawless accentuation. However, there is more that can be done than just analyzing parts of speech. A good prosody model makes guesses about what the listener already knows and deemphasizes that information [3]. Shallow semantic analysis may be used to identify contrast. Consider these examples: "I gave the book to John." (Not to someone else.) "I gave the book to John." (Not the photos.) "I gave the book to John." (Not someone else.) The acoustic side of accents and prosodic boundaries are phone and pause durations, and pitch. Acoustic realization of prosody is highly speaker-dependent, and to some degree domain / application-dependent. Modeling emotion and attitude is a particular challenge. Speaker-specific rule sets learned automatically from relevant data sets seem to be the most appropriate approach. |
|
|  | | mia V.I.P.
Сообщений : 184
Репутация : 7
 | mia | :: Чт Мар 05 2009, 20:56 |
Чт Мар 05 2009, 20:56 | |
| Recording and labeling challenges Creating a new TTS voice quickly is enabled by automation, where possible. Voice recordings, however, cannot be automated since it involves a speaker talking in real time. However, careful planning is needed for selecting the right material to record [4], specifying strict recording conditions and processes, and establishing a process for quality assurance. This is important, in particular if the recording process is being farmed out to different studios. A well designed process assures accurate and efficient reading of the material. It also assures highly consistent recording conditions across all recording sessions of the speaker. Automatic phonetic labeling [5] is a crucial component in Unit-selection based TTS, because its accuracy strongly influences the quality of the synthesized speech. Forty hours or more of recorded speech may have to be labeled. If hand-labeling is considered at all, methods are needed that select the portion of the corpus that would benefit most from hand-labeling. A similar bootstrapping method for labeling prosody has been suggested in [6]. Note that it is usually necessary to adapt the recognizer / automatic labeler to the target speaker and to the recording environment. UNIT SELECTION challenges Unit selection [7-12] is the process of automatically choosing the optimal units from a speech inventory database, given the input text and the added information generated by the front-end. This process usually employs a Viterbi-search that minimizes "target" and "join" cost components. The join cost represents the acoustic mismatch between two recorded units, toward the goal of smooth unit concatenations. The target cost captures the mismatch between the predicted unit specification (phoneme name, duration, pitch, etc.) and actual features of a candidate recorded unit. Challenges in Unit Selection include finding better spectral distance measures that incorporate human perception. An optimal distance measure would rank a set of transitions between available units the same way human listeners do [13, 14]. Also, whether to split the task of Unit Selection into multiple stages (eg, whether to do linguistic-symbolic preselection first, followed by acoustic / spectral selection), and how to handle a large set of candidate units in real time or better, are all topics of ongoing research. Speech signal processing challenges One important result of using Unit Selection for speech synthesis is the reduced need for signal processing. In principle units selected from a very large inventory can be concatenated using minimal signal processing at the boundaries. However, this assumes all necessary units are in the inventory. There is statistical evidence that it may not be practical to expect full coverage [15]. For these cases it seems appropriate to explore optimal signal processing techniques for natural-sounding duration, pitch, volume, and spectral modifications. Since several hours of voice recordings go into a Unit Selection speech inventory, speech compression tech - niques are of interest that encode the speech at transparent quality. Special challenges for speech coders designed for TTS systems are the need for random access (traditional speech coders do not require this capabilty), and the abi - lity to perform signal modifications at the decoding stage. Creating new voices out of a set of existing voices is another challenge. Existing speaker transformation techniques still lack high quality [16]. Using a large number of existing voice inventories, signal processing techniques might be used to create new voices from them. Finally, signal analysis techniques are needed to evaluate recordings objectively, for example, to determine whether a voice talent has become inconsistent with already accepted recordings (due to a cold, fatigue, etc.) and has to be excused from a recording session [17]. TTS integration challenges As TTS becomes ubiquitous, it will have to run on many platforms of various sizes (CPU speed, RAM, disk space). This creates a downward pressure on voice database size (less so on channel density). Consequently, there will be interest in solving the problems of reducing the size of databases without reducing quality (section 7). Running counter to this is Moore's law that over the longer term should loosen some of the restrictions on database size. Still, thinking about the problem today, it's not clear when household appliances with 1GB of storage dedicated to TTS will be common. So for the foreseeable future compression or pruning of databases will be a hot topic. Another aspect of the commoditization is that there will be a shift of emphasis towards making TTS easy to integrate into applications. SAPI for Windows is just the first step. The Java Media Framework is not easy to use, and, in the Unix world, the X window system only covers video. There is hope that within a few years TTS can become an integral part of various operating systems and as a consequence be more seamlessly integrated into applications. For this it is necessary to examine and, where necessary, strengthen or extend existing standards. TTS evaluation challenges How to evaluate TTS systems appropriately is still a largely unsolved research problem [18]. However, it is clear that standardization efforts such as VXML [19] and SALT [20] help in allowing swapping different TTS systems into an existing application. Competitive bench - marking right in a user's application is key for making the optimal buying decision. Passing the Turing test for ever more complicated input texts could be viewed as the ulti - mate evaluation goal for TTS. Consequently, for evalu - ating an individual module of a TTS system, any output that makes the Turing test fail should count as an error. 10. Conclusions This paper attempts to summarize the state-of-the-art and identify the next "hot topics" in TTS Research. It does not provide solutions to any of these open challenges, however. Instead, we try to support the notion that TTS Research still has a long way to go before delivering the perfect-sounding speech output for any input text, with any intended (perhaps subtle) emotional undertones, and in any application. Until then, customizing TTS to do well in restricted domains / applications seems to be a possible line of attack. Even there, research is needed to optimize processes to drive down costs and time-to-market. References - Sproat, R., Black, A., Stanley Chen, S., Kumar, S., Ostendorf, M., and Richards. C., "Normalization of non-standard words, "Computer Speech and Language, 15 (3), pp.287-333, 2001.
- Yarowsky, D. "Homograph Disambiguation in Speech Synthesis. "In: J. van Santen, R. Sproat, J. Olive and J. Hirschberg (eds.), Progress in Speech Synthesis. Springer - Verlag, pp. 159-175, 1996.
- Hirschberg, J., "Accent and discourse context: assigning pitch accent in synthetic speech, "Proceedings of the Eighth National Conf. on Artificial Intelligence, pp. 952-957, 1990.
- van Santen J., Buchsbaum A., "Selecting Training Text via Greedy Rank Covering, "Proc .. 7th ACM-SIAM Symposium on Discrete Algorithms, 1996.
- Wightman, C., and Talkin, D., "The Aligner: A system for automatic time alignment of English text and speech ", Document version 1.7, Entropic Research laboratory, Inc., 1994.
- Strom, V., "From Text to Prosody without TOBI," Proc. ICSLP, Denver, 2002.
- Hunt, A., and Black, A., "Unit Selection in a Concatenative Speech Synthesis System Using a Large Speech Database, " IEEE-ICASSP-96, Atlanta, Vol. 1. pp. 373-376, 1996.
- N. Campbell and A. Black,. "Prosody and the selection of source units for concatenative synthesis, "In: J. van Santen, R. Sproat, J. Olive, and J. Hirschberg, editors, Progress in speech synthesis, pp. 279-282. Springer Verlag, 1996.
- Black, A., and Taylor, P., "Automatically clustering similar units for unit selection in speech synthesis, "Eurospeech, Rhodes, Greece, pp. 601-604, 1997.
- Conkie, A., "Robust unit selection system for speech synthesis, "Joint Meeting of ASA, EAA, and DAGA, Berlin, Germany, 15-19 March, 1999.
- Beutnagel, M., Mohri, M., and Riley, M., "Rapid Unit Selection from a Large Speech Corpus for Concatenative Speech Synthesis, "Proc. Eurospeech, Budapest, Hungary, Sept. 1999.
- Conkie, A., Beutnagel, M., Syrdal, A., Brown, P., "Preselection of candidate units in a unit selection-based text-to - speech synthesis system, "Proc. ICSLP, Beijing, China, 2000.
- Syrdal, A., "Phonetic Effects on Listener Detection of Vowel Concatenation, "Eurospeech, Aalborg, Denmark, 2001.
- Stylianou, Y., and Syrdal, A., "Perceptual and objective detection of discontinuities in concatenative speech synthesis, " Proc. ICASSP, Salt Lake City, UT, 2001.
- van Santen, J., "Combinatorial Issues on Text-to-Speech Synthesis, "Eurospeech, Rhodes, Greece, 1997.
- Stylianou, Y, Cappe, O., and Moulines, E., "Continuous Probabilistic Transform for Voice Conversion, "IEEE Proc. on Speech and Audio Processing, Vol.6, No.2, March 1998, pp.131-142.
- Stylianou, Y., "Assessment and correction of voice quality variabilities in large speech databases for concatenative speech synthesis, "ICASSP, Phoenix, AZ, 1999.
- http://www.slt.atr.co.jp/cocosda/synthesis/evaltext.html
- http://www.voicexml.org
- http://www.microsoft.com/speech
|
|
|  | | | A perspective on the next challenges for TTS research | |
| A perspective on the next challenges for TTS research |
---|
| |